Directors' Blog

Bon voyage Kyle Higham, our latest PhD graduate
Congratulations to Te Pūnaha Matatini PhD student Kyle Higham, our much admired and highly active TPM Whānau past-chair and member, who successfully defended his PhD thesis recently.
Kyle undertook his PhD at the Victoria University of Wellington, researching knowledge diffusion and the dynamics of citation networks under the supervision of TPM investigators Adam Jaffe, Michele Governale and Uli Zuelicke.
Well that’s that out of the way! pic.twitter.com/2V97xYPY20
— Kyle Higham (@SpeckOnADot) November 7, 2018
Always a popular figure at TPM gatherings, Kyle now leaves us to take up an exciting role at the prestigious Ecole polytechnique fédérale de Lausanne (EPFL) in Switzerland.
Well done for all that you’ve achieved Kyle, and thank you for all your work with TPM Whānau. We hope to see you back in the future. Ka kite anō, kia kaha!
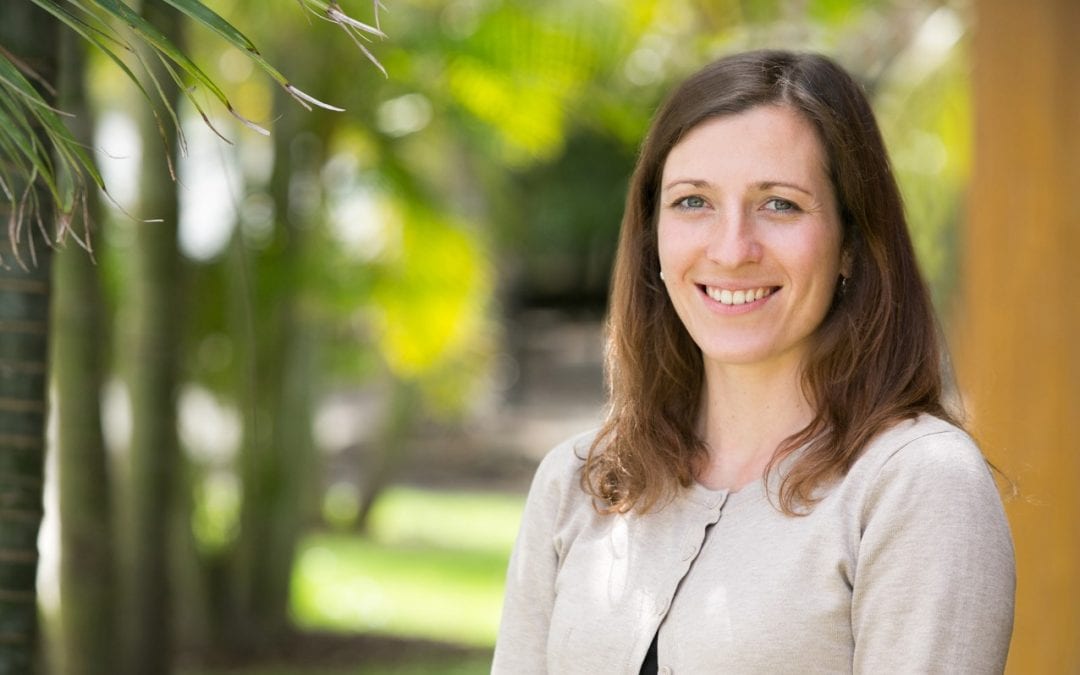
Audrey Lustig presents at major global ecology conference
Dr Audrey Lustig, Associate Investigator at Te Pūnaha Matatini and postdoctoral researcher with the Geospatial Research Institute (GRI) Toi Hangarau, University of Canterbury, presented her work at the 2018 British Ecological Society (BES) held in Birmingham, UK, in December.
Audrey, whose research is focused on spatial modelling of species distribution, presented her paper on regional pest control at the conference, the second largest annual meeting for ecologists in the world. With more than 500 talks and 200 poster presentations, there was an international flavour, which really added to the diversity of speakers, topics, systems and organisms discussed.
BES promotes diversity, equity, access, and inclusion
Audrey said it was a privilege to attend the three-day event. “This really is an exciting place to be for those partial to thinking about the natural world…. It was an incredibly stimulating and well-organised three days, with a lovely balance between unstructured (social) time and scientific talks and posters.”
The BES has taken a national leadership role in promoting diversity, equity, access, and inclusion in science and academia, reflected by a various new initiatives meeting diversity targets across gender, race, and sexual orientation during the event. This included a 1:1 gender ratio at the plenary session, a ‘women in science’ networking session, a LGBT+ and Trans mixer, gender neutral toilets, a ‘meet the plenary speaker for early career researchers’ session, and more.
Conference presentation highlights and challenges
“The wonderful plenary sessions by Samuel M. ‘Ohukani‘ōhi‘a Gon, III on using the Hawaiian Isles as a Model for Biocultural Conservation and by Danielle Lee on cultivating a generation of scholar in your communities were a major highlight of the academic program,” said Audrey.
“I urge people to view their talks when they become available through the BES [website]. We also all had a good laugh when Ken Thompson started listing some of the most inspirational ecology papers of the year. I will remember that ‘gardening is the perfect antidote to thinking that one year’s field data means anything at all!”
“I somehow muddled through my presentation on regional pest management in the invasive species oral session,” Audrey added. “Unfortunately, I felt like a bit of a zombie that day as my body still insisted that it was night time! On the positive side of things, I interacted with so many great scientists. I finally had the opportunity to meet up with Guillaume Latombe and Tim Blackburn and catch up with the incredible Jane Catford and Stéphane Boyer. Lots of cool research opportunities and potential collaborations, so I’m already looking forward to the next British Ecological Society Meeting!”
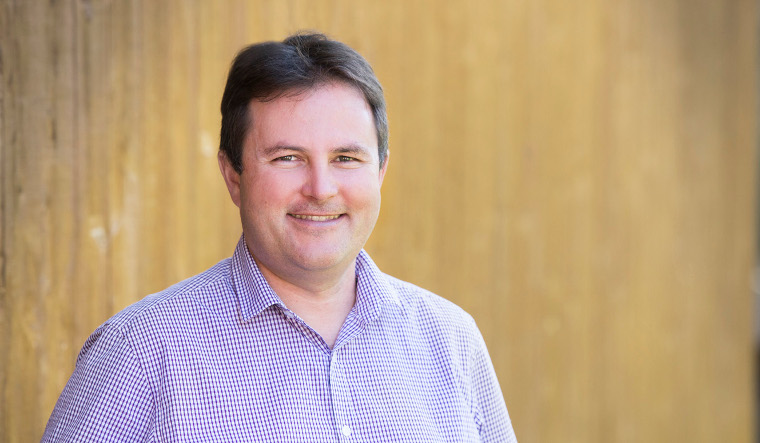
Shaun Hendy appointed to Callaghan Innovation Board
Shaun Hendy, Professor of Physics at the University of Auckland and Director of Te Pūnaha Matatini, has been appointed to the Board of Directors for Callaghan Innovation. Shaun’s appointment was announced recently by the Minister for Research, Science and Innovation Hon Dr Megan Woods.
Shaun will sit on the Callaghan Board for a term of 3 years. Other new appointments announced by Dr Woods include Jennifer Kerr, who is on the board of New Zealand Trade and Enterprise, and sitting members Stefan Korn, George Gong and Robin Hapi, who have had their terms extended.
“Callaghan Innovation is New Zealand’s innovation agency, supporting Kiwi businesses to innovate and collaborate,” says Dr Woods. “They have been major partners in the development of the R&D Tax Incentive which will encourage even more New Zealand firms to undertake R&D activity.”
Te Pūnaha Matatini is delighted with the news of Shaun’s appointment and wholeheartedly congratulates all of the new appointees.

Te Pūnaha Matatini farewells Samin Aref
Congratulations to Samin Aref, a highly valued member of the TPM Whānau, for handing in his PhD thesis recently. Samin commenced his PhD under the supervision of TPM Investigator Mark Wilson, working on computationally intensive problems in complex networks. Samin has the honour of being the first ever TPM intern to graduate with a PhD.
Another reason to celebrate is that Samin has secured a post-doctorate position at the prestigious Max Plank Institute for Demographic Research in Rostock, Germany.
Samin flew out to Europe earlier this week. However, not before we were able to give him a proper send-off – during the TPM Whānau Retreat in Ōtaki and subsequently at TPM HQ in Auckland. Samin’s supervisor Mark Wilson and our director Shaun Hendy gave speeches recognising his contributions during his time with TPM.
Kia kaha, all the best in Germany Samin! Stay in touch and we hope to see you back in Aotearoa in the future!
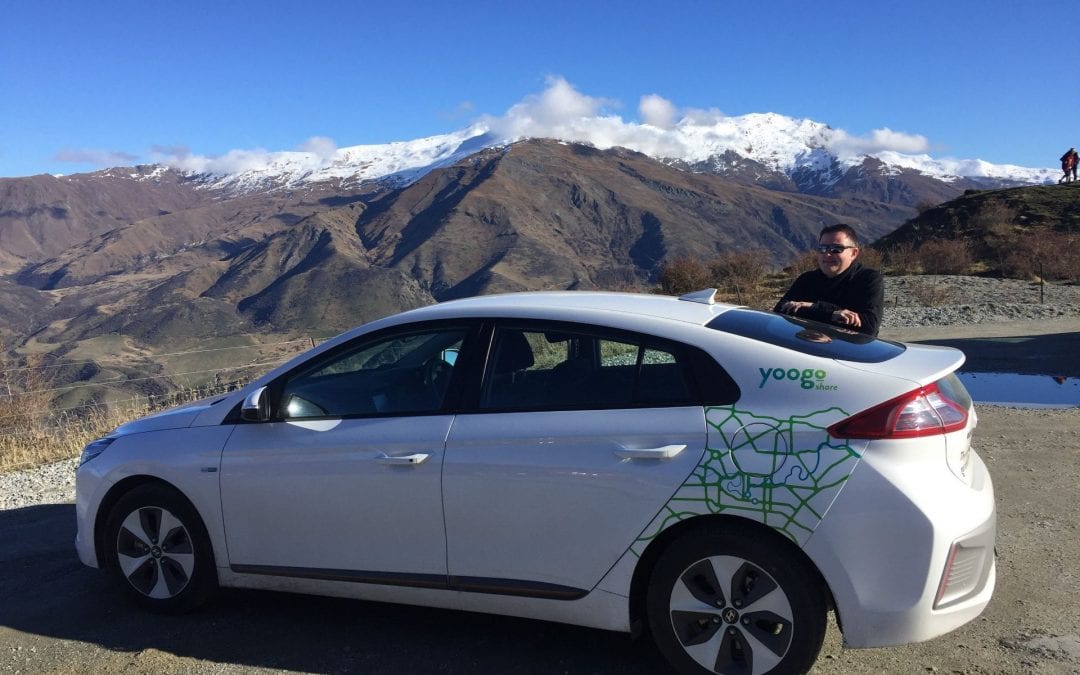
Shaun Hendy #nofly2018 update
As many of you will be aware, our director Shaun Hendy has been travelling a lot differently this year. Despite being a frequent domestic and international traveller, Shaun decided that for 2018 he would set an example and highlight his concern about climate change by not using air travel for the entire calendar year. The hashtag #nofly2018 was born and, from the first day in January, he has walked the talk – effectively turning his back on flying as a means to get around, favouring instead modes of land transport that emit less carbon.
Various news outlets have covered Shaun’s journey over the year, including Radio New Zealand and the New Zealand Herald. So, now that we’re into September, how is he getting on? Well, very impressively according to the stats. By mid-September 2017, Shaun had made 10 return flights from Auckland to Wellington, at a cost of 2.66 metric tonnes of CO2 (equivalent)*. So far this year he has been to Wellington and back six times (once by car, three times by train, and twice by bus) at a cost of just 0.458 metric tonnes of CO2 (equivalent)*. What is more he’s got more done in Wellington this year: in 2017 his 10 flights gave him 10 business days in Wellington, while in 2018 he has had 21 working days down the capital.
Not flying has its advantages
In addition to reducing his carbon footprint, Shaun says one of the great advantages to taking it slower by road or rail is that you can actually get a lot of work done on the way and plan in more meetings with investigators in one trip. Te Pūnaha Matatini, a national Centre of Research Excellence, has investigators spread across New Zealand.
“Over the last two weeks [for example] I have travelled #nofly2018 style from Auckland to Queenstown and back again,” said Shaun. “It was great to catch up with a number of investigators on the way through. I spent a beautiful sunny day at the University of Canterbury, catching up with [Te Pūnaha Matatini investigators] Alex James, Jeanette McLeod, Mike Plank, and Audrey Lustig, as well as dropping by to see Rebecca Turner at Scion. The conversations that day were very timely as I have gotten involved with MPI’s Mycoplasma Bovis Eradication Science Advisory Group to help them think about how they can use the various data sets they have at their disposal.”
Electric vehicles becoming more feasible
On his most recent trip, Shaun was also sponsored by Yoogo Share, an electric vehicle share company that has 100 electric vehicles based in eight locations in Christchurch.
“They lent me one of their Hyundai IONIQ’s for five days,” said Shaun. “I had about 1,000km to drive, including the odd hill or two. The IONIQ doesn’t yet have the range of a petrol vehicle. Depending on the terrain, you’ll get around 100-160 km between charges, although running the heater will shave 10-15% off this. A fast charge takes around 15 minutes and will boost your battery up to about 80% capacity, but if you’ve got another 10 minutes or so you can charge it up to 95%.”
Luckily, there is a growing network of charging stations across New Zealand, which means electric vehicle users can get to most places without too much trouble.
“From Christchurch, I charged up at Geraldine, then Tekapo, followed by a big charge at Twizel to make sure I made it over the Lindis pass, and then a final top up in Cromwell,” said Shaun. “The IONIQ was great to drive – I had no problems taking it up over the Crown Range Rd. Definitely give it a go next time you are in Christchurch.”
Follow Shaun on Twitter for #nofly2018 updates!
Keep up-to-date with Shaun Hendy’s travels on Twitter by following the #nofly2018 hashtag.
Heading back to Christchurch today in the @yoogo_nz Hyundai IONIQ. It’s been a great climate-friendly few days #nofly2018 pic.twitter.com/sleS3kUEBI
— Shaun Hendy (@hendysh) August 19, 2018
*Calculated using the Enrivo-Mark Travel Emissions Calculator.
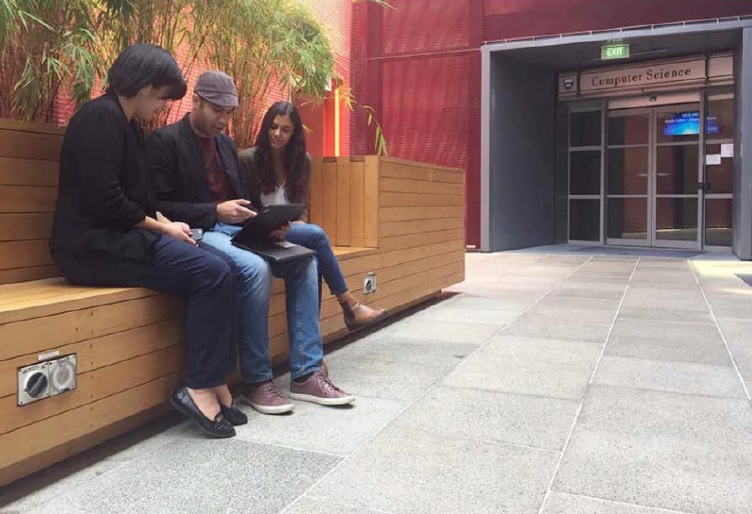
Interns work to enhance use of te reo Māori
In the summer of 2016-2017, Te Hiku Media and Te Pūnaha Matatini co-funded a number of student internships – work from which led to the development of Kōrero Māori – a project to teach machines how to speak te reo Māori.
One of the interns was Jamie Chow, a conjoint BComm/BEng (Honours) degree student from the University of Auckland. Jamie’s work on Te Hiku’s Data Analytics and Visualisation Project involved using online audience data to measure the performance of the organisation’s digital platform, matching it with other information such as demographics and geographical data.
Internship leads to ongoing employment opportunity
Following his 10-week summer internship, Jamie continued working on the project for Te Hiku in part-time employment over the course of 2017.
“We kept Jamie on board,” says Te Hiku’s R&D Scientist and Engineer Keoni Mahelona. “He had the internship then we hired him on contract for about 6 months or so. Just casually, furthering the work that he did for that project. That was really useful.”
Jamie was able to create visualisations of the data that allowed Te Hiku staff to quickly understand and interpret the behaviour of their audience. Staff can now immediately examine their content for audience engagement, allowing them to adapt their future programming.
“The project mainly involved developing tools to obtain usage data for their online platform and present this data in a suitable form to their staff, producers, and content creators,” says Jamie.
“My project was very self-driven so it was always challenging and engaging. It was clear even from the beginning that I would learn a lot throughout the project. Te Hiku Media seemed to be pleased with the results and I’m happy they’re using and benefiting from my work.
“Overall, it was a great experience and I’m grateful that I had the opportunity to work with some awesome people.”

Jamie Chow, Te Pūnaha Matatini 2016-17 summer student intern (centre), with Keoni Mahelona, Te Hiku Media (left) and Shaun Hendy, Te Pūnaha Matatini (right).
About the Kōrero Māori project
Te Hiku’s Data Analytics and Visualisation Project led to discussions about the need for ICT tools for te reo Māori. Working together, Keoni’s team developed the Kōrero Māori project to create computer language models for te reo Māori – the basic tools which will allow machines to be able to transcribe and speak the language.
Keoni is optimistic about how Te Hiku and Te Pūnaha Matatini can collaborate in terms of research direction in the future.
“I do look forward to us continuing to work together… and doing research around Te Reo, language processing and also looking to the languages of the Pacific – because there’s a lot of similarities,” says Keoni. “If we can turn these into machine models, perhaps we can learn something about the evolution of our languages.
Keoni says that Te Pūnaha Matatini has been very helpful and open in terms of how the data are managed.
“It’s about managing data in a way that aligns with our tikanga and our values, as Māori and as a Māori organisation and as indigenous people. Our language is our culture. It’s our identity. We’re talking about giving that to a machine and I guess the question comes, well, who owns that data or who owns the machines that have access to that data,” explains Keoni.
“As indigenous people, we want to maintain some sovereignty… and Te Pūnaha Matatini has been helpful in terms of having quite a broad and open understanding of those aspects of the project.”

Students thrilled with summer internship experiences
Students who’ve taken part in previous summer internship programmes run by Te Pūnaha Matatini have expressed a high level of satisfaction with their experiences. Indeed, the 10-week paid internship programme provides an excellent opportunity for students to hone their data analytics skills while working for organisations in a real-world setting.
A total of 21 undergraduate and postgraduate university students from around New Zealand were selected for our 2017-18 programme. Divided into teams, the interns were placed on a wide range of projects working for various organisations, including Iwi, government and private firms based in either Auckland or Wellington.
There were some exciting new opportunities. One team, for instance, were placed on a project with Dragonfly Data Science and Te Hiku Media based in Wellington. Their internship involved work related to Te Hiku’s Kōrero Māori project, developing language tools that will enable speech recognition and natural language processing of te reo Māori. This requires the collection of more than 100,000 sentences and 250 hours of Māori language corpus. Once complete, it aims to provide these language tools to the Māori ICT industry.
Interns share their thoughts and details of their work
One of the student interns on this project was William Asiata, a BSc Mathematics graduate from the University of Canterbury and a current Master of Information Technology student at the University of Auckland.
“As a result of the internship I was able to generate a corpus of all te reo Māori spoken in Parliament which will be included in the greater corpus used to train the digital natural language processor language model,” said William. “As an interesting by-product we also produced some statistics about the historical usage of te reo in Parliament. I had the opportunity to learn and practice the Python and R programming languages and exercise data processing skills.
“I believe that it was a great opportunity for an inexperienced student to sharpen one’s skill set, to clarify future career goals, and to gain direct insight into the ICT and data science industries through practical work experience on meaningful, high-impact projects and the chance to learn directly from working professionals,” he added.
Check out our latest guest blog! This one is by maths grad William Asiata about his internship with @dflydsci, @TeHiku and @PunahaMatatini, working on the #KōreroMāori project & analyzing the rapid recent growth in the use of te reo by NZ parliamentarians. https://t.co/oxI0kuoPMp pic.twitter.com/POhuQ6Q9fQ
— Te Pūnaha Matatini (@PunahaMatatini) March 1, 2018
Another team worked on a project supporting research by Kate Hannah, Te Pūnaha Matatini’s Executive Manager, into the historical representation of women in science.
Emma Vitz, a statistics and psychology graduate from Victoria University of Wellington assigned to this project, researched an algorithm that classifies people by gender according to their first name, and blogged about the ethical pitfalls of such an approach. Emma also began research into networks underlying science collaboration in New Zealand. “I particularly enjoyed using both R and Python in the internship, and collaborating with researchers and other interns from Te Pūnaha Matatini,” said Emma.
Comp programs that learn from past experience/data are all in vogue. However, as data science consultant @EmmaVitz explains, such machine learning can reinforce racism when crunching population-based numbers. Check out her timely & thought-provoking piece: https://t.co/IKYI6juu7X pic.twitter.com/c6kEe8wVwI
— Te Pūnaha Matatini (@PunahaMatatini) February 1, 2018
Also on the team was Beth Rust, a BA (Hons) history graduate from Victoria University of Wellington, who conducted a literature review of the background and achievements of women in science.
“Women are everywhere in science,” said Beth. I noticed a few trends: a lot of early women scientists tended to be in botany – then later women dominated home science – now they are everywhere. I’ve also learnt a lot these past ten weeks, not just in terms of the history of science but also in a more general sense,” she added. “I’m very grateful for the experience and everything it’s taught me.”
It was a privilege hosting history grad Beth Rust over the summer. Check out her blog – an in-depth investigation into the extensive contributions of Māori & Pacific women in science over the years & the importance of their representation within the field. https://t.co/op9fZSXnSh pic.twitter.com/9rv31a86iz
— Te Pūnaha Matatini (@PunahaMatatini) February 11, 2018
Te Pūnaha Matatini Whanau member Stephen Merry, who is pursuing a PhD in mathematics at the University of Canterbury, also took part in the internship programme working with the Social Investment Agency in Wellington.
“I worked on two projects,” said Stephen. “The first investigated the scope of data held inside and outside of the Integrated Data Infrastructure, and the second examined how people’s use of health services is affected by the services’ accessibility. This internship gave me the opportunity to work in a different environment, and I felt a genuine sense of purpose completing the projects,” he added. “My colleagues in the Social Investment Agency were enormously helpful and understanding throughout, and the experience overall is something I would recommend to anyone interested.”
Following the programme, interns were invited to blog about their work for the Te Pūnaha Matatini website and these articles resulted in very positive feedback on Twitter – with even some New Zealand parliamentarians chiming in!
We love hearing about this stuff! 🤓One of the lesser-known perks of hosting a large publicly-available text corpus is seeing the cool projects that come out of it. (For those playing along at home, you can find #Hansard reports on our website: https://t.co/h8GdImYvud) #nzpol https://t.co/s2RaMq0hrN
— NZ Parliament (@NZParliament) March 8, 2018
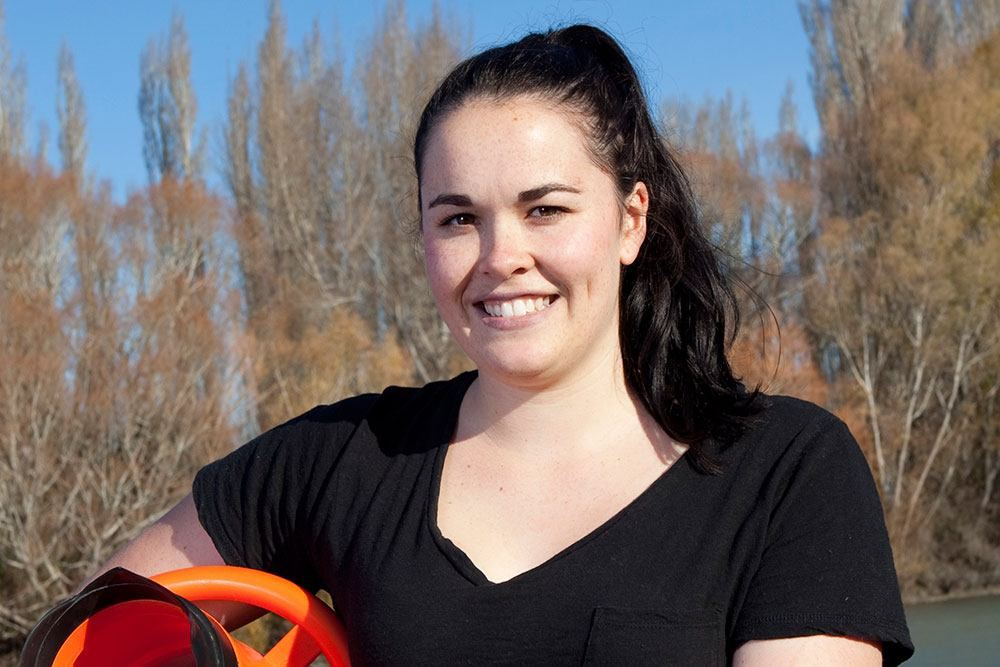
Project to boost scientist mātauranga capability
A Te Pūnaha Matatini research project that aims to improve the way in which scientists connect and work with Māori has been awarded $100,000 in funding by New Zealand’s Ministry of Business, Innovation & Employment (MBIE).
The project, part of MBIE’s Te Pūnaha Hihiko: Vision Mātauranga Capability Fund, will be led by Dr Tara McAllister (pictured above), an environmental scientist with the University of Auckland, in collaboration with ecologist Dr Cate Macinnis-Ng and earth systems scientist Dr Daniel Hikuroa, Principal Investigators with Te Pūnaha Matatini at the University of Auckland. Importantly, the project team will partner with Mahaanui Kurataiao Limited, an environmental and resource management advisory firm based in Canterbury.
While there are some excellent examples of scientists engaging well with Māori communities, there are also instances when connecting has been a struggle.
“We want to look at how we make those interactions more successful, more productive, and more workable for everybody involved,” Dr Macinnis-Ng says.
“So we are going to co-develop a project with an Iwi group, where we’ll look at what their science needs are, and work out who in our field can deliver those things. By co-developing the project, it’s all about what the needs are of that group, rather than imposing what scientists want to do.”
The project will be conducted in a reflective way so the project team can understand what works best for the different groups involved. It will also develop te reo science materials appropriate for school curricula.
“We’ll be developing some teaching materials for kura kaupapa to make science more accessible to everyone,” says Dr Macinnis-Ng.
The project will be very important to Te Pūnaha Matatini’s wider research programme, says Shaun Hendy, the Centre’s Director and Professor of Physics at the University of Auckland.
“Building close engagement with Māori communities and learning about the mātauranga of complex systems is a wonderful opportunity for us,” he says.
“Not only will this project be essential to us in meeting our research goals, it will also provide social, economic, and environmental benefits to Aotearoa New Zealand.”
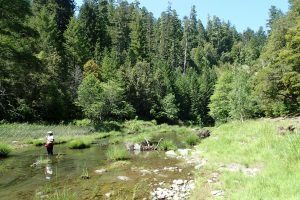
Project lead Dr Tara McAllister (Te Aitanga a Mahaki) out in the field. Tara has a strong research focus on freshwater ecology and management.
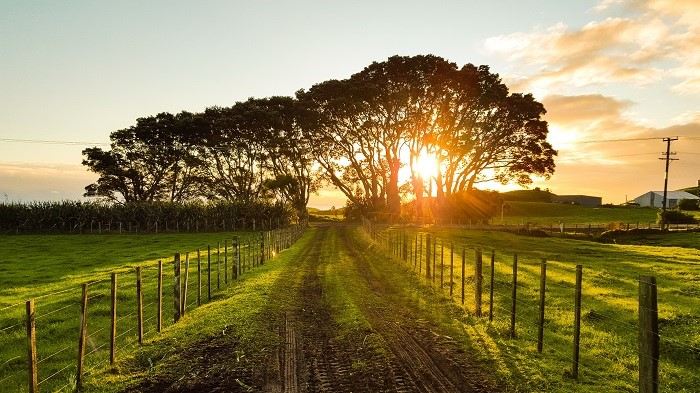
Testing large-scale predator control in Hawke’s Bay
New Zealand has an excellent record of conserving its native flora and fauna through pest control measures, especially in large uninhabited areas. Predator Free 2050 is a bold initiative that aims to rid the country of its most damaging invasive predators. However, to completely eliminate such predators from our shores, new and ambitious approaches are needed.
Implementing effective predator control over large areas
New Zealand’s unique and diverse native species of flora and fauna are extremely vulnerable to invasive mammals. Our often-publicised successes in conserving the country’s biodiversity by managing pests has mainly been restricted to large uninhabited areas. Meanwhile, large tracts of land owned by private individuals remain relatively unprotected.
When it comes to land management decisions such as pest control actions, careful negotiations are required with a wide range of stakeholders with differing views – from cat-lovers to rabbit-haters – so that agreements can be reached.
Experience has shown there are minimum thresholds for landholder participation in predator control measures for them to be successful. In practice, coordinated community efforts are required so that pest reinvasion from a few untreated properties does not compromise pest control achieved by others.
Another crucial element is biological connectivity between properties – the establishment of ‘safe passage’ corridors crossing landowner boundaries greatly assists in the dispersal of native species between fragments of suitable habitat. Large-scale pest control is therefore a spatial issue with social, environmental, and economic components.
The spread model is still being developed to provide more functionality for managers. In particular, we are investigating the ways in which landholders influence one another, how agencies influence landholders, and the presence of key influential landholders who might help catalyze actions are the current focus of research. Ultimately, the aim of the model is to improve strategic planning for mammal control at regional scales. Also, this model serves as a template for future dynamic maps of other mammal species.
Large-scale Cape to City research project in Hawke’s Bay
Te Pūnaha Matatini investigators Audrey Lustig, Mike Plank and Alex James, from the University of Canterbury, are involved in a large-scale predator control initiative covering 26,000 hectares of agricultural land in Hawke’s Bay, part of a wide range of research activities referred to as the Cape to City research project by the Hawke’s Bay City Council.
“This is just a start for a much more ambitious project that proposes a vision to eliminate invasive predators from the entire country,” says Audrey. “In this work, we develop a generic modeling approach as a planning tool for predicting the abundance and the likely persistence of four New Zealand top mammalian predators in the light of potential changes in management effort across human-dominated landscape.”
The first part of the project aims to generate a computer model for predicting the distribution and abundance of mammalian species across the landscape, the ways in which animals move from their natal sites, and how their distributions and abundance are affected by control interventions.
Such modelling can help inform managers on the likelihood of success of a specific pest control action (assuming every landholder participates in the control action). It also allows exploration of some of the mechanisms by which mammal populations might recover after control operations.
Importance of multi-stakeholder engagement
The work builds on a pre-existing knowledge base and data acquired by the Hawke’s Bay Regional Council, Department of Conservation, Manaaki Whenua and the Biological Heritage Challenge to bring about practical improvements in mammalian pest management in New Zealand.
“Such inter-organisational joint effort is common in New Zealand, but to me, what was critical was to bring a more practical insight into my research,” says Audrey. “In particular, the provision of direct feedback from decision-makers forms an integral part of the learning process and enriches my research experiences and outcomes, while providing useful information to the Hawke’s Bay Regional Council.”
For further details about this project, please contact us today.
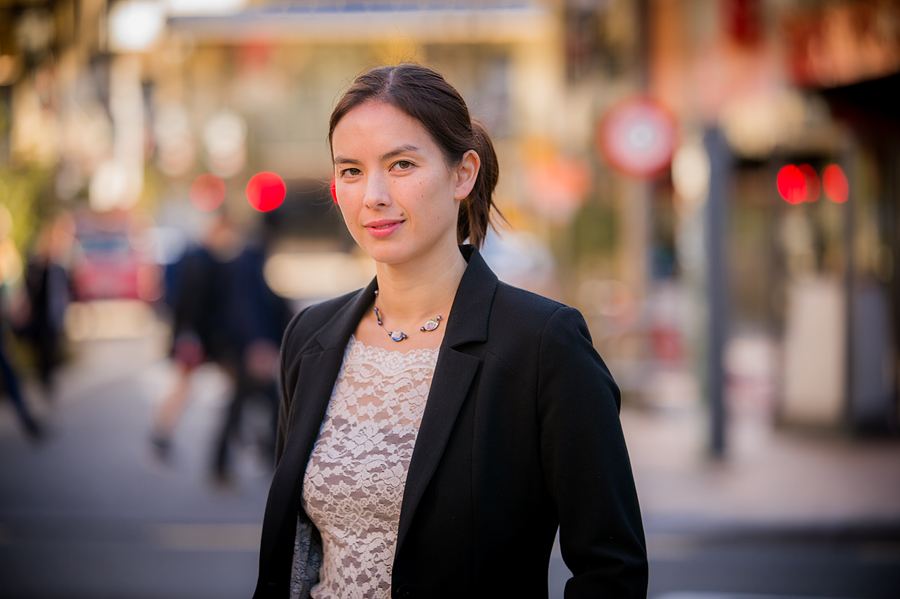
New report shows mothers take pay cut to have a baby
A new report co-authored by Dr Isabelle Sin, Te Pūnaha Matatini Principal Investigator from Motu Economic and Public Policy Research (pictured), has revealed that mothers experience an average 4.4% wage decrease after having a baby.
The report’s findings made the front page of the New Zealand Herald print edition, with commentary from Associate Professor Siouxsie Wiles – also a Te Pūnaha Matatini Principal Investigator – and her husband and mathematician Professor Steven Galbraith, both from the University of Auckland. Check out the article here. Isabelle Sin was also interviewed on RNZ’s Nine To Noon – listen in here.